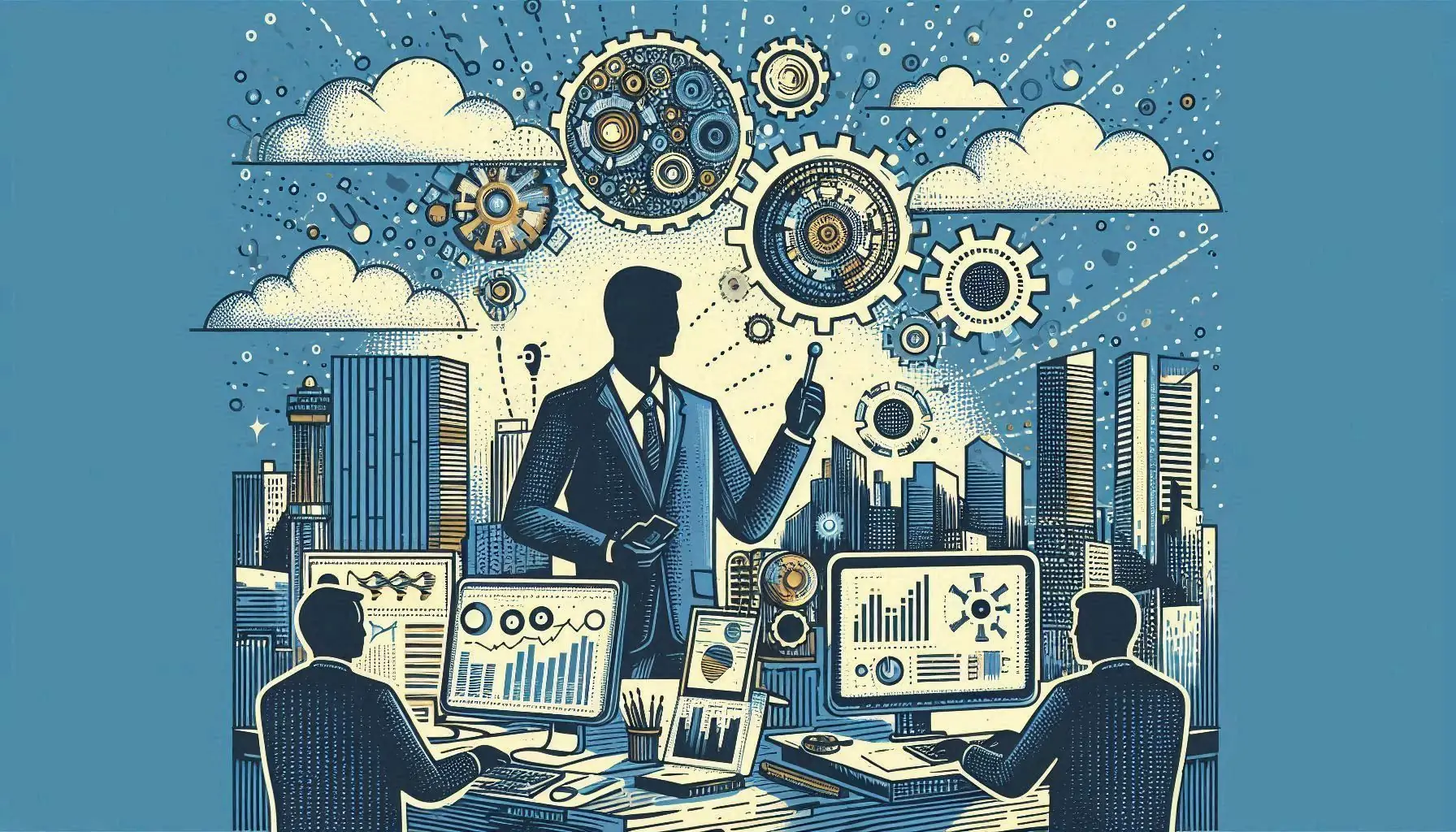
How Machine Learning Is Revolutionizing Business Operations
Most decision-makers are aware that the machine learning (ML) age is here, and it arrived less than 100 years after the concept was first posited as a mathematical model.
The opportunities it unlocks in a business context are tough to quantify because of the wide range of appealing applications and use cases.
So to simplify things, let’s break down just a few examples of the areas in which operations can be overhauled by ML.
Machine Learning in Supply Chain Optimization
ML is taking supply chain management to new heights by optimizing operations and driving efficiency. Here’s how:
Demand Forecasting
Accurate demand forecasting is crucial for inventory management. ML models analyze historical sales data, market trends, and external factors to predict future demand accurately.
Benefits include:
Reduced stockouts, which cost companies around $1 trillion collectively
Minimized excess inventory
Enhanced customer satisfaction
Enhanced Delivery
ML makes efficient delivery route planning a possibility for organizations of all sizes, all but guaranteeing timely deliveries and cost savings. It does this by analyzing traffic patterns, weather conditions, and road networks to determine the most optimal routes in real time.
The advantages of this are:
Decreased fuel consumption
Lowered transportation costs
Improved delivery times
Inventory Management
ML augments inventory management by predicting reorder points and preventing overstocking or understocking situations.
There are several strategies involved, such as:
Real-time stock monitoring using sensors
Automated replenishment orders based on predictive analytics
Supplier Risk Assessment
Assessing supplier reliability becomes simpler with ML. Models evaluate various risk factors like financial health, geopolitical events, and past performance to identify potential disruptions in the supply chain. Since these can cost companies 6-10% of yearly earnings, the benefits of minimizing them are obvious.
Advantages include:
Proactive mitigation of risks
Strengthened supplier relationships
Warehouse Operations Optimization
From automating picking processes to streamlining warehouse layout designs, ML improves warehouse efficiency remarkably. This is something giants like Amazon have embraced, and smaller companies are following suit.
Operational benefits to mention are:
Reduced manual errors
Faster order fulfillment times
Enhancing Customer Experience with ML Algorithms
Machine learning significantly elevates the customer experience by providing personalized and efficient services. This is something 80% of people expect from the brands they interact with, so again there’s little room for falling short. Here’s how businesses are leveraging ML:
Personalized Recommendations
ML algorithms analyze user behavior, preferences, and purchase history to generate tailored recommendations.
Benefits worth mentioning include:
Increased sales conversions
Enhanced customer satisfaction
Higher retention rates
Customer Support Automation
AI-driven chatbots and virtual assistants use natural language processing (NLP) to understand and respond to customer inquiries efficiently.
This leads to:
Round-the-clock support availability
Quick resolution of common issues
Reduced operational costs for customer service teams
Sentiment Analysis
Businesses employ sentiment analysis to gauge customer emotions from reviews, social media posts, and feedback forms.
The practical applications of this include:
Identifying areas for product improvement
Responding proactively to negative sentiments
Enhancing overall brand reputation
Dynamic Pricing Strategies
ML models predict optimal pricing strategies based on factors such as demand fluctuations, competitor pricing, and seasonal trends.
The impact of this factors in:
Maximized revenue potential
A competitive edge in the market
Churn Prediction & Prevention
By analyzing patterns in user activity, ML algorithms identify customers at risk of churning, allowing businesses to implement targeted retention strategies.
Outcomes here are:
Improved loyalty programs
Tailored re-engagement campaigns
Automating Routine Business Processes via AI Systems
Automation through AI systems streamlines routine business processes, enhancing efficiency and reducing human error. Here’s how it’s done:
Robotic Process Automation (RPA)
RPA utilizes bots to handle repetitive tasks such as data entry, invoice processing, and payroll management. Businesses are already spending over $10 billion on this, and further investment is anticipated.
The benefits of note are:
Significant time savings
Decreased operational costs
Minimized manual errors
Document Processing
AI-powered document processing extracts and interprets information from various formats like PDFs, emails, and scanned images.
Key advantages include:
Faster data retrieval
Improved accuracy in information handling
Reduced reliance on paper-based processes
Workflow Optimization
AI algorithms optimize workflows by identifying bottlenecks, predicting workload peaks, and suggesting improvements for resource allocation.
The impact of this is:
Enhanced productivity levels
Smoother operation flows
Better resource utilization
Customer Relationship Management (CRM)
AI integration in CRM systems helps automate customer interactions, track communication history, and provide actionable insights for sales teams. This is helping such platforms deliver an ROI of at least 211%, thus justifying adoption.
Operational benefits can be:
Personalized outreach campaigns
Increased lead conversion rates
Strengthened customer relationships
Automated Reporting & Analytics
Automated reporting tools compile data from various sources, generate detailed reports, and offer predictive analytics to guide decision-making processes.
Outcomes include:
More informed strategic decisions
Time-efficient report generation
Improved data visualization capabilities
Wrapping Up
These examples of what machine learning can do for businesses in need of an operation glow-up are compelling on their own, but really shine when combined. And as mentioned they are not only available to major corporations, so smaller firms that want to reap the rewards just need to get to work on embracing ML tools.